Ethics of Machine Learning — Can we trust computers to be unbiased?
And ways of combating bias in Machine Learning and Artificial Intelligence.
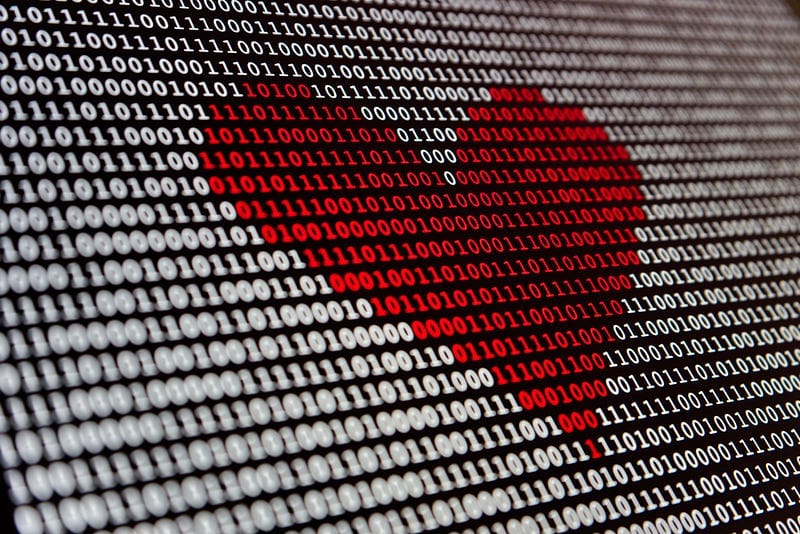
You’d think that a computer wouldn’t be able to have a bias since it can’t have feelings or emotions. However, you’d be wrong to assume that a computer wouldn’t be able to learn biases. Machine Learning (ML) models and Artificial Intelligence (AI) have been researched for many years, and their capabilities have drastically improved. Machine Learning has given us a lot of benefits such as more accurate predictive text when texting on phones and better language translation systems that can handle plain text and text in images. However, there are still obstacles that need to be tackled to ensure high-quality algorithms including learned racial biases. In 2019, IBM published a piece on their Developer’s site addressing this issue of machine learning and bias.
Some of you may be coming here as experts in Machine Learning ready to learn and explore more in-depth the intersection of machine learning and racial injustice. In that case, feel free to skip to the next paragraph. Others of you may have stumbled here by accident and have only heard “Machine Learning” as a buzzword thrown around in the news or on the internet. To those of you who don’t already know what Machine Learning is, here’s a brief explanation. Machine Learning is a technique that was developed and researched in the 90s and has only become popular within the past 10 years, and it will continue to rise in the future. The main goal of ML is that a computer will be able to, given a set of data (images to categorize, sentences to sort, etc), learn the patterns within the data to predict a similar but new set of data (a different set of images with the same categories, new sentences to sort, etc.). The algorithm’s effectiveness is largely determined by how the training and validation occur and what the dataset contains. Machine Learning is also used in Artificial Intelligence systems to help AI learn as well.
Three years ago, the Wall Street Journal published an article in which they reported that facial recognition software using face detection algorithms was largely racially biased in the same way that one would think humans were. Even before that, in 2015, one of Google’s face detection algorithms tagged 2 black American users as gorillas. Of course, there are many ways someone could explain this. Either the classification algorithm for images of different classes genuinely had trouble discerning what groups of dark-colored pixels were gorillas and people, or there was a lack of diverse skin tones among the images that were in the “people” category. You can assume, that it was probably the latter and not the former and that the dataset wasn’t diverse enough to represent the diverse human population.
With all of these problems with ML, you might be wondering whether there are easy ways to tackle these sorts of problems. There are, in fact here’s a list of some very simple things that can help avoid these biases. I’ll explain what each of these means later.
- Intentionally un-bias the training and validation data
- Ask a third party to evaluate your datasets
Let’s look at the first option.
Intentionally un-bias the training and validation data.
What does that even mean? When you want a Machine Learning algorithm to learn from the “real world” using “real world” data, it’s going to inherently learn human biases. This is especially true for AI and ML which are trained on data that is produced naturally. Other cases where the bias creeps in are via unconscious bias during the creation of the dataset itself. On image classification datasets, especially in the west, the pictures of people that are included in the datasets are more likely to be largely white in demographic. It is less likely that a dataset will include an even mix of diverse people. Making sure that the dataset itself is unbiased, is one effective way to combat bias in machine learning and AI. Another method to help ensure that models are not imbalanced and biased is to utilize model report cards, as introduced by researchers at the University of Toronto. These model report cards accurately indicate what types of data are preferred by a certain model. This is very much like the nutrition facts of a specific machine learning algorithm.
Another way that ML models and AI can be trained ethically is to have a third party evaluate the datasets and algorithms. Twitter has a Health Data team that is dedicated to ensuring the algorithms built by their developers are ethical and unbiased. Having a third-party look at the data gives an outside perspective that can ensure that underlying hidden biases are not being enforced. These Health Data teams look at the datasets being used to train these algorithms and ensure that the datasets aren’t producing results exacerbating pre-existing social biases. They check the datasets and the parameters of the model itself to see if any modifications can be made to improve the performance of these ML algorithms.
Now, you might be thinking about whether the companies that are building these ML and AI systems are actually enforcing these standards. You might be wondering about accountability. This is an ongoing concern for those who might be doubting the effectiveness of ML and AI and reducing biases of all kinds — racial, gender, and others. There have been plenty of articles published within the past year such as this one on how biased AI perpetuates racial injustice or this one on this one California city banned predictive policing.
Every time one of these articles comes out, I’m always curious as to what kind of data was used to train these algorithms. In terms of predictive policing, machine learning can only use historical data to predict the future, so it only makes sense that the algorithms perpetuate existing bias patterns because these racial biases have been ingrained in the data for so many years. The only way to actively un-bias these models would be to either come up with fake data to feed the computer or use enough examples of positive outcomes for low-income and minority neighborhoods — in the case of predictive policing. Especially since predictive policing has been shown to target low-income and minority neighborhoods as typically active hotspots for crime. Predictive policing is a practice that uses algorithms and historic data to predict which areas need more policing. Since high crime rate areas are historically in low-income and predominantly black neighborhoods, this practice carries some inherent biases. Rather than breaking the cycle of bias, predictive policing enhances it and makes it stronger since it will always suggest the same neighborhoods as probable locations for the crime.
Considering all of this, you might still be concerned about the effectiveness of Machine Learning. Is it really as great as it’s made out to be? In most cases, yes. ML and AI are very good at making working with big data more efficient and can help speed up a lot of very repetitive tasks. In other cases, such as predictive policing and other areas where any amount of bias is harmful, ML might not be the best way to do things. ML is only a tool that can be used to either help or harm people. Hopefully, it’s mainly used to help people. There currently isn’t too much regulation on where ML and AI can be used, but there are some legal considerations. More research is needed to ultimately understand the inner workings of ML and AI, and maybe then will we be able to tackle these bias issues.
This blog post was previously submitted as an assignment when I took Science Communication @ Cornell University during the Fall 2020 semester.